8 mins to read
CESAR .
Publicado em: 31 de maio de 2023
From doubting GenA.I.’s worth to championing it for ability to co-create and boost productivity
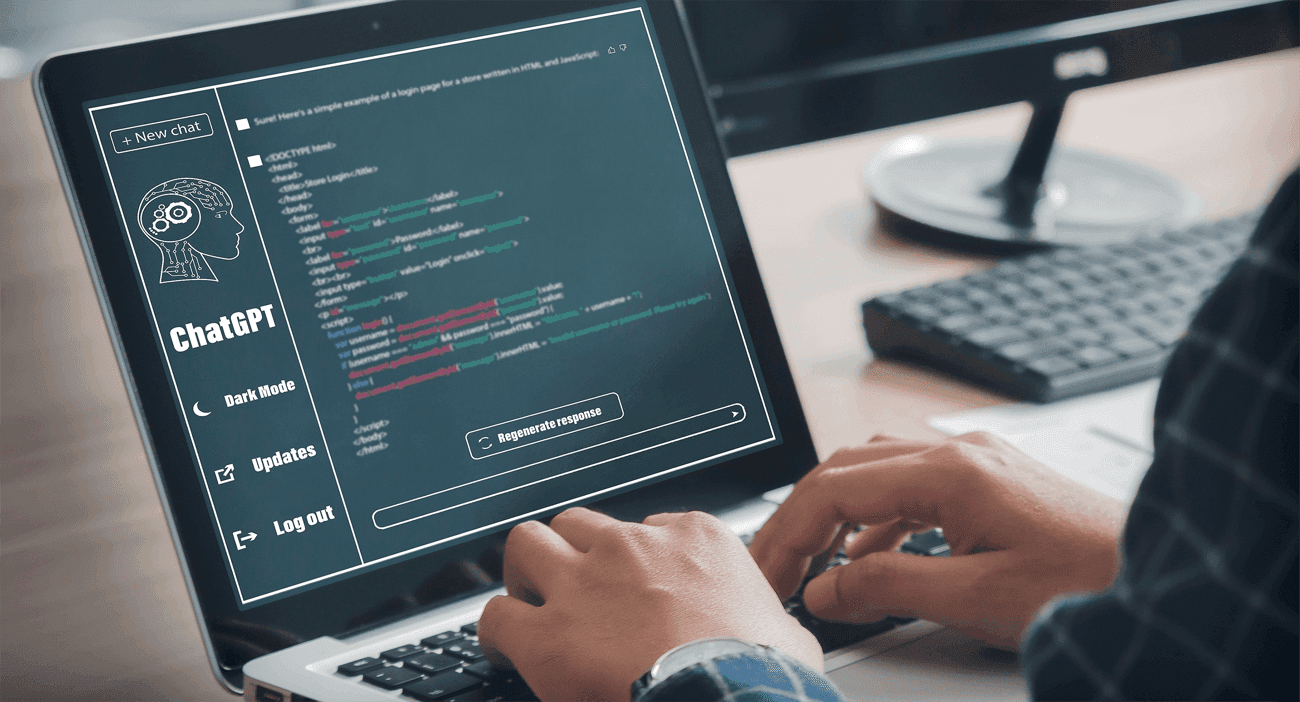
In a remarkable turnaround, Fábio Maia, the head of systems engineering at CESAR, transformed from being a skeptic to becoming a staunch advocate of generative artificial intelligence in the workplace. This fast-emerging subset of A.I. can create groundbreaking content based on human speech prompts, potentially revolutionizing the way we work and create.
Maia’s initial skepticism – fueled by A.I.’s checkered history – labeled GenA.I. as a mere buzzword: an overhyped technology that could never live up to the grandiose promises made by its advocates. But a recent renaissance in A.I. technology that catapulted several A.I. models into the world spotlight and common lexicon prompted him to give GenA.I. a new shot, leading to mind-blowing results that could forever change the landscape of creativity and efficiency in his eyes.
These benefits, Maia found, did not come without their faults and trials, but despite the myriad hidden challenges in GenA.I.’s usage, Maia now stands as a staunch proponent for GenA.I.’s supporting role in the co-creation of exceptional works that surpass what any one expert could achieve on their own.
Experimenting with code generation using GenA.I.
Embarking on his journey with cautious optimism, Maia fed the GenA.I. system – in this case ChatGPT – a few parameters, eager to witness its capabilities. The results were nothing short of astonishing: the GenA.I. tool not only comprehended Maia’s admittedly sparse instructions, but it managed to generate usable code that precisely fulfilled his intended request.
CESAR’s Fábio Maia is a new convert to GenA.I.’s ability to speed up coding
Maia, a man accustomed to the more manual, “old way” of coding – who explicitly used to write the exact instructions a computer should follow to complete a task – marveled at ChatGPT’s ability to grasp the nuances of his queries. “I was genuinely impressed by how well it seemingly understood what I asked from it,” he recalled. But while extraordinary, Maia drew parallels to historical shifts in computing, underscoring that this type of change is one we’ve already seen.
“We’ve had this kind of shift before. In the beginning, when we were just a level above machine language, with its binary and hexadecimals, you needed to write these things (code) out in assembly. One actually needed to remember the opcodes and operands to get a computer to do anything. Then came compilers, able to generate code based on instructions that more closely resembled human speech – which enabled us to write code at a higher level of abstraction. Back then, people worried about technology replacing jobs, as compilers required fewer people than were needed with assembly, but that’s not what happened.” – Maya explained.
“It’s like memory in computers,” he continued, “the more memory you add, the more uses people find for that memory. That’s what has been happening with software for decades. You raise the abstraction level, and we were able to do much more with less code; but then you have much more demand for increasingly advanced software.”
Nearly 40 percent increased productivity per engineer
Today, Maia believes that GenA.I.’s effectiveness within the workplace will go far beyond writing simple, “boilerplate” code. In fact, Maia estimates that GenA.I. tools can be used to expedite up to about 40 percent of an engineer’s daily workload. He emphasized that most software engineers spend their time extending or fixing existing systems, rather than writing code from scratch. And this is where, he believes, GenA.I. truly shines.
When faced with unfamiliar codebases, libraries, or frameworks consisting of thousands, if not millions, of lines of code, the typical workflow can involve hours of scouring through databases, search engines, and online forums to understand what each piece of code does. But now, armed with GenA.I., Maia finds himself skipping the search and diving straight into the answers.
The system’s conversational nature allowed him to ask questions in natural language and receive prompt responses.
Generative A.I. provides a powerful ally in this endeavor by streamlining the operation and speeding up the process of exploring codebases, libraries, and pre-existing code. For example, rather than tracing variables through each line of a code system to diagnose a problem, Maia can now simply ask the GenA.I. to add comments to each line to explain to him what the code is doing, and it responds with relatively good answers in a fraction of the time it might take a human.
“It’s like having the support of a giant team of low-level coders. You have to double check because it gets things wrong, but the simple, repetitive tasks that require less knowledge can be done quickly and automatically.” – Maia shared.
Coding’s shorter feedback loops make it an ideal for GenA.I.
“Because coding is more deterministic and highly structured, we see results faster”, Maia explained. “With medicine,” he offered as a counterexample, “you describe symptoms of your disease, and ChatGPT might give you a diagnosis and a prescription. Because the feedback loop on biology can be slower, it might take a long time before you realize that medicine is actually making you worse. With code, we have compilers and type checkers and unit tests, so you know nearly instantly if the model got it wrong.”
This quick, iterative nature of coding makes it a perfect pair for optimization with GenA.I. tools. All these improvements culminate in a significant reduction in time spent searching through search engines and forums, which lets engineers focus on more problem-solving tasks and innovation.
IT leaders agree GenA.I. will have a transformative impact
Maia isn’t alone in extolling GenA.I.’s potentially transformative impact on the world. Google CEO, Sundar Pichai, recently said that “A.I. is one of the most important things humanity is working on. It’s more profound than, I don’t know, electricity or fire.” One survey conducted by Salesforce, as another example, even found that 57 percent of more than 500 senior IT leaders surveyed consider GenA.I. to be a “game changer,” with 67 percent prioritizing GenA.I. within the next 18 months.
Navigating the caveats
Yet, Maia emphasizes the importance of cautious adoption and expertise in using GenA.I. tools. “While they are formidable assets in the hands of knowledgeable professionals, reliance on them without a deep understanding of the subject matter can lead to erroneous outcomes,” he said.
He raises critical questions about ensuring individuals possess the necessary experience to discern between accurate and flawed outputs and notes that ongoing education and training are crucial for success. Further, he warns overuse or improper use of these tools could cause skill deficits in areas where fledgling engineers do not put in the time to learn certain basic concepts via experience; instead relying on the A.I. to do all the smaller tasks without first understanding the core concepts they are shortcutting. That will hit you back hard as soon as outcomes deviate a little bit from the expected. And will prevent you from properly guiding the AI in the first place when it comes to non-trivial systems.
Looking ahead, Maia envisions a future where generative A.I. becomes an indispensable co-creator that collaborates with human ingenuity to redefine the software engineering landscape. He advocates for a symbiotic relationship, where the strengths of humans and machines merge to unlock unparalleled creativity and innovation. “The possibilities are vast, but responsible development, transparency, and ethical considerations must guide our path forward,” he said.
“I even envision that at the big companies, you will be able to talk to your code. Let’s suppose that I have an issue reported by the field that something has happened with the system: a failure. If your entire code base is incorporated in a GenA.I. model, instead of having to stop to think on where this could be happening in the code and conducting experiments to confirm, you could just ask the model where the problem is, and the GenA.I. will point you to the most promising areas to look at.” – Maia shared.
Summing it up
In conclusion, Maia’s journey from doubting the worth of GenA.I. to championing its co-creation for software coding and other types of work highlights the truly transformative nature of this technology today, with its capabilities and resulting impacts only growing as the technology continues to evolve rapidly. While initially skeptical, his experimentation with GenA.I. revealed its remarkable ability to comprehend complex instructions and generate usable code.
He emphasizes that GenA.I. excels in assisting software engineers by streamlining the process of working with existing codebases, reducing search time, and enabling faster problem-solving, but also acknowledges the importance of cautious adoption and the need for expertise to discern accurate outputs.